AI Art Part 1: How it works
If you have any involvement with the furry fandom – and if you're reading this, you probably do – you will have seen a lot of talk about Artificial Intelligence (AI) over the past few weeks/months, particularly about AI-generated art. What you'll also have seen is that most of this talk has been characterised by fear and anger. Several furry websites and organisations, such as Inkbunny, Fur Affinity and the Furry Writers' Guild, have all issued statements or updated their policies to ban AI-generated content or aspects thereof.
As a largely artistic community and given that AI-generated content threatens the status quo, this is completely understandable. The objections to AI art have been numerous; some rely on nebulous and abstract concepts such as AI art being emotionless, some consider it unfair to artists who have put effort into learning their skills and others have chosen to focus on the economic aspects of competing against AI. Anti-AI sentiment has grown in the art community and spilled off of the internet in the form of legal challenges against several companies involved in providing AI art services.
It would be out of scope of this text to address every single argument for or against AI. Instead, I would like to focus on two aspects of the debate; one which is objective and one which is subjective. The first, objective, aspect is what AI is and how it works. This is important because it's difficult to have a proper discussion about the technology when it is misunderstood – and I think that most discussion around AI fundamentally misunderstands how it works. The second, subjective, aspect involves copyright and the ethics of training AI. This is a question about the sort of world in which we wish to live and how we should treat one another. I feel that these two questions are the most important ones for deciding how AI will fit into our world. Hopefully, I can correct some misconceptions about how AI works and put forward a view of the world which others will find appealing as well.
Due to the length of this article, it has been split in two. The second part will address the social and ethical aspects of AI-generated art.
Thank you to 'Yote, who has a PhD in computational biology, for providing feedback and fact-checking for this article.
Mathematical models do not store copies of artwork
One of the major misconceptions about AI models is that they contain copies of the training artwork. For example, the Stable Diffusion Litigation page claims:
Stable Diffusion contains unauthorized copies of millions—and possibly billions—of copyrighted images.
If that were true, it would be an amazing advance in compression technology. To quote machine learning scientist Daniel Lowd:
Stable Diffusion starts with 100 terabytes of images and produces a model that fits in 4 gigabytes -- that's a compression factor of 25,000, like turning a 1MB image into 40 bytes. So it's simply not capable of remembering very much from any particular image.
— Daniel Lowd (@dlowd) January 4, 2023
There are no unauthorised copies of people's artwork inside the AI models. What the models actually store is information about patterns, probabilities and relationships in the training data. For example, if one trained an AI model on the phrase "The quick, brown fox jumped over the lazy dog." It would link the noun "dog" with the adjective "lazy." If that model were then asked to describe a dog, it would return the phrase "lazy dog."
If more sentences were used in the training data, it would pick up other associations. For instance, the model could additionally be trained on the following sentences:
"Rover is a good dog."
"Cujo is a rabid dog."
"Rex is a lazy dog."
In that case, it would, again, not store those exact sentences, but the associations between the individual words. The model would thus know that the noun "dog" is associated with various adjectives, as well as how often those adjectives are used; good (1), rabid (1), lazy (2). If one asked this more complex model to describe a dog, it would describe it as "lazy" half the time but as either "good" or "rabid" the other half of the time. (If you want to see how these associations can result in ChatGPT telling you a completely made-up fact, this Twitter thread gives an example. Don't rely on AI for the truth.)
In reality, these models are, of course, more complicated. "Dog" would not only be associated with different adjectives but also with different verbs, moods, sentence structures and so on. To illustrate these complexities with a real-world example that I am more familiar with, let us consider protein sequences. A protein sequence can be represented as a string of letters. Many organisms share the same proteins but with slight variations in their sequences.
Below, one can see the sequences of a protein from five different organisms. There are many positions where they are identical, as well as many positions where they differ.
Seq_1 VTALAAEIQGIEKASHVKDKLVEMVRLSETSYACGVACSAE
Seq_2 ATMLAAEYTGVEKVPHIQEKIIDMVRLSETSHACAIASAMR
Seq_3 AAMLAAEYNGANRASHIKDKLIEMMSTAELAYACGIACSAE
Seq_4 AAAVAADYNGCERMAHIRDKIVEMSSTAELAYGCGIACSAE
Seq_5 VTALAAEANGIQKVAHVREKLTEFASTAELAYAAGIASAVF
cons .: :**: * :: .*:::*: :: :* ::...:*.:
Often, biologists want to find specific proteins out of hundreds or thousands of different sequences. Because the sequences are not exactly the same, this is not as easy as matching the letters. One way to solve the problem is to construct a mathematical model which represents the protein sequence that the biologist would like to find. The most common kind of such a mathematical model is called a Hidden Markov Model (HMMs), and is used in many other examples of machine learning as well. In an HMM, each position in the sequence will be represented by different numbers which describe the likelihood of a particular letter being at that position. The complete HMM for the above sequences can be seen below.
HMER3/f [3.3.2 | Nov 2020] NAME AI_example LENG 41 ALPH amino RF no MM no CONS yes CS no MAP yes DATE Sat Jan 21 11:15:36 2023 NSEQ 5 EFFN 1.958008 CKSUM 768265948 STATS LOCAL MSV -7.3844 0.71942 STATS LOCAL VITERBI -7.8331 0.71942 STATS LOCAL FORWARD -4.3077 0.71942 HMM A C D E F G H I K L M N P Q R S T V W Y m->m m->i m->d i->m i->i d->m d->d COMPO 1.77460 3.56289 3.37654 2.54932 3.88692 2.85134 3.44426 2.66192 2.87595 2.61967 3.41321 3.58160 4.14161 3.65185 3.28496 2.58476 2.90111 2.54501 5.64837 3.56973 2.68618 4.42225 2.77519 2.73123 3.46354 2.40513 3.72494 3.29354 2.67741 2.69355 4.24690 2.90347 2.73739 3.18146 2.89801 2.37887 2.77519 2.98518 4.58477 3.61503 0.01490 4.60991 5.33226 0.61958 0.77255 0.00000 * 1 1.07655 4.51423 4.54944 4.18072 4.17960 3.71781 4.93370 2.66553 4.09564 2.99028 4.08016 4.17769 4.39660 4.35755 4.29279 3.16539 3.26297 1.29139 5.82172 4.62651 1 a - - - 2.68618 4.42225 2.77519 2.73123 3.46354 2.40513 3.72494 3.29354 2.67741 2.69355 4.24690 2.90347 2.73739 3.18146 2.89801 2.37887 2.77519 2.98518 4.58477 3.61503 0.01490 4.60991 5.33226 0.61958 0.77255 0.48576 0.95510 2 1.29694 4.48588 4.20265 3.91721 4.69816 3.32862 4.82967 3.91735 3.88852 3.75730 4.63301 3.87776 4.11365 4.15857 4.12317 2.79850 0.96197 3.41800 6.09830 4.93414 2 t - - - 2.68618 4.42225 2.77519 2.73123 3.46354 2.40513 3.72494 3.29354 2.67741 2.69355 4.24690 2.90347 2.73739 3.18146 2.89801 2.37887 2.77519 2.98518 4.58477 3.61503 0.01490 4.60991 5.33226 0.61958 0.77255 0.48576 0.95510 3 1.14120 4.53946 4.24526 3.80891 3.86270 3.71210 4.58751 2.96546 3.68482 2.66818 1.62213 3.96595 4.31382 3.98867 3.91748 3.12144 3.21735 2.78854 5.48390 4.28986 3 a - - - 2.68618 4.42225 2.77519 2.73123 3.46354 2.40513 3.72494 3.29354 2.67741 2.69355 4.24690 2.90347 2.73739 3.18146 2.89801 2.37887 2.77519 2.98518 4.58477 3.61503 0.01490 4.60991 5.33226 0.61958 0.77255 0.48576 0.95510 4 3.70244 4.98243 5.48182 4.97189 3.48005 5.00405 5.45475 2.34680 4.80311 0.64946 3.26175 5.16092 5.19845 4.87821 4.86370 4.41788 3.94772 1.71958 5.63431 4.56585 4 L - - - 2.68618 4.42225 2.77519 2.73123 3.46354 2.40513 3.72494 3.29354 2.67741 2.69355 4.24690 2.90347 2.73739 3.18146 2.89801 2.37887 2.77519 2.98518 4.58477 3.61503 0.01490 4.60991 5.33226 0.61958 0.77255 0.48576 0.95510 5 0.33153 4.75021 4.40686 4.30261 4.98231 3.54503 5.20354 4.24369 4.34423 4.07997 5.06373 4.20507 4.35385 4.61429 4.49324 3.11385 3.44352 3.74593 6.31351 5.23450 5 A - - - 2.68618 4.42225 2.77519 2.73123 3.46354 2.40513 3.72494 3.29354 2.67741 2.69355 4.24690 2.90347 2.73739 3.18146 2.89801 2.37887 2.77519 2.98518 4.58477 3.61503 0.01490 4.60991 5.33226 0.61958 0.77255 0.48576 0.95510 6 0.33153 4.75021 4.40686 4.30261 4.98231 3.54503 5.20354 4.24369 4.34423 4.07997 5.06373 4.20507 4.35385 4.61429 4.49324 3.11385 3.44352 3.74593 6.31351 5.23450 6 A - - - 2.68618 4.42225 2.77519 2.73123 3.46354 2.40513 3.72494 3.29354 2.67741 2.69355 4.24690 2.90347 2.73739 3.18146 2.89801 2.37887 2.77519 2.98518 4.58477 3.61503 0.01490 4.60991 5.33226 0.61958 0.77255 0.48576 0.95510 7 3.43492 6.12237 1.68728 0.74334 5.40323 3.57512 4.19263 4.97795 3.30231 4.43167 5.31456 3.02712 4.24146 3.36947 3.96374 3.27628 3.74092 4.51212 6.55860 4.99378 7 e - - - 2.68618 4.42225 2.77519 2.73123 3.46354 2.40513 3.72494 3.29354 2.67741 2.69355 4.24690 2.90347 2.73739 3.18146 2.89801 2.37887 2.77519 2.98518 4.58477 3.61503 0.01490 4.60991 5.33226 0.61958 0.77255 0.48576 0.95510 8 2.06451 4.41970 4.27522 3.74192 3.25732 3.97043 4.23304 2.01640 3.61473 2.50817 3.52375 3.94814 4.37080 3.86221 3.81471 3.28593 3.17242 2.51230 4.84466 1.43810 8 y - - - 2.68618 4.42225 2.77519 2.73123 3.46354 2.40513 3.72494 3.29354 2.67741 2.69355 4.24690 2.90347 2.73739 3.18146 2.89801 2.37887 2.77519 2.98518 4.58477 3.61503 0.01490 4.60991 5.33226 0.61958 0.77255 0.48576 0.95510 9 2.91151 5.22016 2.94098 2.59707 4.63518 3.55773 3.91446 4.09211 2.66971 3.63242 4.43600 1.41687 4.05263 2.19415 3.10531 2.90512 2.12187 3.69417 5.79956 4.43094 9 n - - - 2.68618 4.42225 2.77519 2.73123 3.46354 2.40513 3.72494 3.29354 2.67741 2.69355 4.24690 2.90347 2.73739 3.18146 2.89801 2.37887 2.77519 2.98518 4.58477 3.61503 0.01490 4.60991 5.33226 0.61958 0.77255 0.48576 0.95510 10 3.81492 5.45729 4.62640 4.63584 5.65376 0.15643 5.64356 5.49443 4.90777 5.02078 6.04315 4.79423 4.85917 5.17511 5.00568 4.01542 4.34608 4.88236 6.49628 5.79945 10 G - - - 2.68618 4.42225 2.77519 2.73123 3.46354 2.40513 3.72494 3.29354 2.67741 2.69355 4.24690 2.90347 2.73739 3.18146 2.89801 2.37887 2.77519 2.98518 4.58477 3.61503 0.01490 4.60991 5.33226 0.61958 0.77255 0.48576 0.95510 11 2.21690 2.47093 4.97639 4.42650 3.69636 4.34360 4.85134 1.31569 4.28634 2.52367 3.60633 4.50479 4.71044 4.48222 4.39065 3.69433 3.34039 1.47482 5.37891 4.18445 11 i - - - 2.68618 4.42225 2.77519 2.73123 3.46354 2.40513 3.72494 3.29354 2.67741 2.69355 4.24690 2.90347 2.73739 3.18146 2.89801 2.37887 2.77519 2.98518 4.58477 3.61503 0.01490 4.60991 5.33226 0.61958 0.77255 0.48576 0.95510 12 3.10741 5.65622 2.63136 1.14200 4.97522 3.56298 3.95380 4.47946 2.79392 3.94772 4.74704 2.17386 4.10163 2.07586 3.29682 3.02022 3.36483 4.04517 6.07797 4.62571 12 e - - - 2.68618 4.42225 2.77519 2.73123 3.46354 2.40513 3.72494 3.29354 2.67741 2.69355 4.24690 2.90347 2.73739 3.18146 2.89801 2.37887 2.77519 2.98518 4.58477 3.61503 0.01490 4.60991 5.33226 0.61958 0.77255 0.48576 0.95510 13 3.65274 5.69993 4.25732 3.36314 5.32114 4.17595 3.94128 4.58068 0.88945 3.90410 4.79717 3.68447 4.49461 3.06632 1.29308 3.62207 3.75302 4.27206 5.84309 4.77807 13 k - - - 2.68618 4.42225 2.77519 2.73123 3.46354 2.40513 3.72494 3.29354 2.67741 2.69355 4.24690 2.90347 2.73739 3.18146 2.89801 2.37887 2.77519 2.98518 4.58477 3.61503 0.01490 4.60991 5.33226 0.61958 0.77255 0.48576 0.95510 14 1.70350 4.43860 4.56906 4.02831 3.63350 4.09076 4.61870 2.35180 3.90721 2.45794 2.29720 4.19921 4.51398 4.14214 4.09036 3.43394 3.25144 1.30101 5.28452 4.09722 14 v - - - 2.68618 4.42225 2.77519 2.73123 3.46354 2.40513 3.72494 3.29354 2.67741 2.69355 4.24690 2.90347 2.73739 3.18146 2.89801 2.37887 2.77519 2.98518 4.58477 3.61503 0.01490 4.60991 5.33226 0.61958 0.77255 0.48576 0.95510 15 1.26790 4.54168 3.88385 3.52692 4.75948 3.30666 4.59107 4.19716 3.55753 3.85633 4.65449 3.67550 2.02260 3.83825 3.89290 1.40754 3.06313 3.60011 6.07523 4.86347 15 a - - - 2.68618 4.42225 2.77519 2.73123 3.46354 2.40513 3.72494 3.29354 2.67741 2.69355 4.24690 2.90347 2.73739 3.18146 2.89801 2.37887 2.77519 2.98518 4.58477 3.61503 0.01490 4.60991 5.33226 0.61958 0.77255 0.48576 0.95510 16 4.16383 5.73045 4.20345 4.08613 4.12447 4.32074 0.25450 5.00519 3.93134 4.36771 5.51417 4.44331 4.93209 4.47945 4.12138 4.24824 4.51598 4.72299 5.55036 4.06661 16 H - - - 2.68618 4.42225 2.77519 2.73123 3.46354 2.40513 3.72494 3.29354 2.67741 2.69355 4.24690 2.90347 2.73739 3.18146 2.89801 2.37887 2.77519 2.98518 4.58477 3.61503 0.01490 4.60991 5.33226 0.61958 0.77255 0.48576 0.95510 17 3.54630 4.75235 5.59814 5.14623 4.10344 5.13914 5.91650 0.89339 5.08343 2.51727 3.83695 5.30971 5.37588 5.34536 5.25639 4.58365 3.81248 1.05050 6.23595 5.01354 17 i - - - 2.68618 4.42225 2.77519 2.73123 3.46354 2.40513 3.72494 3.29354 2.67741 2.69355 4.24690 2.90347 2.73739 3.18146 2.89801 2.37887 2.77519 2.98518 4.58477 3.61503 0.01490 4.60991 5.33226 0.61958 0.77255 0.48576 0.95510 18 3.46755 5.63608 3.96022 3.16055 5.19962 4.05885 3.88589 4.49501 1.29674 3.84094 4.70030 3.54159 4.38511 1.93553 1.30234 3.43041 3.59563 4.15883 5.81282 4.68782 18 k - - - 2.68618 4.42225 2.77519 2.73123 3.46354 2.40513 3.72494 3.29354 2.67741 2.69355 4.24690 2.90347 2.73739 3.18146 2.89801 2.37887 2.77519 2.98518 4.58477 3.61503 0.01490 4.60991 5.33226 0.61958 0.77255 0.48576 0.95510 19 3.46214 6.18507 1.01093 1.18983 5.45443 3.57047 4.20477 5.03883 3.34310 4.48425 5.37691 3.01745 4.24761 3.38456 4.02774 3.29290 3.77258 4.56564 6.61533 5.02794 19 d - - - 2.68618 4.42225 2.77519 2.73123 3.46354 2.40513 3.72494 3.29354 2.67741 2.69355 4.24690 2.90347 2.73739 3.18146 2.89801 2.37887 2.77519 2.98518 4.58477 3.61503 0.01490 4.60991 5.33226 0.61958 0.77255 0.48576 0.95510 20 3.87084 5.71858 4.09111 3.63874 5.29113 4.19915 4.43565 4.79502 0.34848 4.21693 5.19083 4.00655 4.68950 3.62245 2.89455 3.89670 4.09159 4.48039 6.06846 5.02983 20 K - - - 2.68618 4.42225 2.77519 2.73123 3.46354 2.40513 3.72494 3.29354 2.67741 2.69355 4.24690 2.90347 2.73739 3.18146 2.89801 2.37887 2.77519 2.98518 4.58477 3.61503 0.01490 4.60991 5.33226 0.61958 0.77255 0.48576 0.95510 21 3.80724 5.02442 5.68951 5.16056 3.48280 5.23184 5.62087 1.23010 5.01230 0.85413 3.24075 5.36819 5.32322 5.01768 5.03835 4.64732 4.03285 2.34407 5.69523 4.66185 21 l - - - 2.68618 4.42225 2.77519 2.73123 3.46354 2.40513 3.72494 3.29354 2.67741 2.69355 4.24690 2.90347 2.73739 3.18146 2.89801 2.37887 2.77519 2.98518 4.58477 3.61503 0.01490 4.60991 5.33226 0.61958 0.77255 0.48576 0.95510 22 3.29372 4.59282 5.16579 4.65246 3.93192 4.67037 5.26009 1.20806 4.54858 2.56260 3.75480 4.80004 4.99692 4.79111 4.71002 4.04686 2.18626 1.14601 5.77807 4.56896 22 v - - - 2.68618 4.42225 2.77519 2.73123 3.46354 2.40513 3.72494 3.29354 2.67741 2.69355 4.24690 2.90347 2.73739 3.18146 2.89801 2.37887 2.77519 2.98518 4.58477 3.61503 0.01490 4.60991 5.33226 0.61958 0.77255 0.48576 0.95510 23 3.43543 6.12887 1.60710 0.77617 5.40784 3.57353 4.19166 4.98346 3.30364 4.43559 5.31848 3.02436 4.24051 3.36840 3.96776 3.27561 3.74154 4.51636 6.56361 4.99555 23 e - - - 2.68618 4.42225 2.77519 2.73123 3.46354 2.40513 3.72494 3.29354 2.67741 2.69355 4.24690 2.90347 2.73739 3.18146 2.89801 2.37887 2.77519 2.98518 4.58477 3.61503 0.01490 4.60991 5.33226 0.61958 0.77255 0.48576 0.95510 24 3.69034 5.00661 5.26811 4.77143 1.95203 4.81965 4.82864 2.69511 4.60097 1.82432 0.91952 4.86653 5.04951 4.63877 4.64499 4.20411 3.92270 2.85770 5.02057 3.61407 24 m - - - 2.68618 4.42225 2.77519 2.73123 3.46354 2.40513 3.72494 3.29354 2.67741 2.69355 4.24690 2.90347 2.73739 3.18146 2.89801 2.37887 2.77519 2.98518 4.58477 3.61503 0.01490 4.60991 5.33226 0.61958 0.77255 0.48576 0.95510 25 2.02672 4.32856 4.11438 3.56220 3.54256 3.77031 4.24685 2.71586 3.46840 2.58327 2.46901 3.79936 4.22095 3.72308 3.70772 2.21441 3.03029 1.53392 5.05474 3.85467 25 v - - - 2.68618 4.42225 2.77519 2.73123 3.46354 2.40513 3.72494 3.29354 2.67741 2.69355 4.24690 2.90347 2.73739 3.18146 2.89801 2.37887 2.77519 2.98518 4.58477 3.61503 0.01490 4.60991 5.33226 0.61958 0.77255 0.48576 0.95510 26 2.95040 5.01430 3.59292 3.10916 4.72646 3.63192 4.11797 4.18677 2.63400 3.72907 4.56922 3.48622 4.18796 3.28527 1.43906 1.19849 3.28687 3.75670 5.83043 4.58299 26 s - - - 2.68618 4.42225 2.77519 2.73123 3.46354 2.40513 3.72494 3.29354 2.67741 2.69355 4.24690 2.90347 2.73739 3.18146 2.89801 2.37887 2.77519 2.98518 4.58477 3.61503 0.01490 4.60991 5.33226 0.61958 0.77255 0.48576 0.95510 27 3.04975 4.65686 4.36594 3.91415 3.68958 3.98684 4.65319 2.74951 3.74019 1.29771 3.60860 4.12753 4.49808 4.06698 3.95665 3.38588 1.26213 2.65769 5.42810 4.23102 27 t - - - 2.68618 4.42225 2.77519 2.73123 3.46354 2.40513 3.72494 3.29354 2.67741 2.69355 4.24690 2.90347 2.73739 3.18146 2.89801 2.37887 2.77519 2.98518 4.58477 3.61503 0.01490 4.60991 5.33226 0.61958 0.77255 0.48576 0.95510 28 0.91773 4.48761 4.14985 3.91234 4.90160 3.27500 4.87896 4.31990 3.95108 4.03545 4.84199 3.85414 4.08383 4.19195 4.19270 1.22072 3.09331 3.65430 6.24951 5.08803 28 a - - - 2.68618 4.42225 2.77519 2.73123 3.46354 2.40513 3.72494 3.29354 2.67741 2.69355 4.24690 2.90347 2.73739 3.18146 2.89801 2.37887 2.77519 2.98518 4.58477 3.61503 0.01490 4.60991 5.33226 0.61958 0.77255 0.48576 0.95510 29 3.86001 5.93253 3.10541 0.31659 5.41784 3.95765 4.69178 5.05532 3.69726 4.56407 5.57407 3.66171 4.61389 3.93455 4.14135 3.79818 4.19080 4.68124 6.42367 5.24820 29 E - - - 2.68618 4.42225 2.77519 2.73123 3.46354 2.40513 3.72494 3.29354 2.67741 2.69355 4.24690 2.90347 2.73739 3.18146 2.89801 2.37887 2.77519 2.98518 4.58477 3.61503 0.01490 4.60991 5.33226 0.61958 0.77255 0.48576 0.95510 30 3.10920 4.67927 4.46825 4.01164 3.62966 4.07307 4.71087 2.70249 3.83076 1.03713 3.52912 4.21610 4.55912 4.13918 4.02921 3.47114 1.55450 2.64217 5.41636 4.23717 30 l - - - 2.68618 4.42225 2.77519 2.73123 3.46354 2.40513 3.72494 3.29354 2.67741 2.69355 4.24690 2.90347 2.73739 3.18146 2.89801 2.37887 2.77519 2.98518 4.58477 3.61503 0.01490 4.60991 5.33226 0.61958 0.77255 0.48576 0.95510 31 0.91773 4.48761 4.14985 3.91234 4.90160 3.27500 4.87896 4.31990 3.95108 4.03545 4.84199 3.85414 4.08383 4.19195 4.19270 1.22072 3.09331 3.65430 6.24951 5.08803 31 a - - - 2.68618 4.42225 2.77519 2.73123 3.46354 2.40513 3.72494 3.29354 2.67741 2.69355 4.24690 2.90347 2.73739 3.18146 2.89801 2.37887 2.77519 2.98518 4.58477 3.61503 0.01490 4.60991 5.33226 0.61958 0.77255 0.48576 0.95510 32 4.23206 5.51800 4.70960 4.46293 2.34906 4.77512 1.90030 4.21340 4.23848 3.45863 4.72933 4.36890 5.12453 4.41296 4.35017 4.19878 4.45428 4.09590 4.01679 0.62915 32 Y - - - 2.68618 4.42225 2.77519 2.73123 3.46354 2.40513 3.72494 3.29354 2.67741 2.69355 4.24690 2.90347 2.73739 3.18146 2.89801 2.37887 2.77519 2.98518 4.58477 3.61503 0.01490 4.60991 5.33226 0.61958 0.77255 0.48576 0.95510 33 0.61310 4.53383 4.15659 3.99646 4.99681 1.88274 4.98315 4.41507 4.10319 4.13875 4.95265 3.91942 4.13172 4.31968 4.31851 2.81416 3.16069 3.73148 6.32458 5.20103 33 A - - - 2.68618 4.42225 2.77519 2.73123 3.46354 2.40513 3.72494 3.29354 2.67741 2.69355 4.24690 2.90347 2.73739 3.18146 2.89801 2.37887 2.77519 2.98518 4.58477 3.61503 0.01490 4.60991 5.33226 0.61958 0.77255 0.48576 0.95510 34 1.63422 0.75910 4.76813 4.54838 4.64841 3.37279 5.13040 3.74009 4.38879 3.69843 4.63964 4.14743 4.18994 4.59739 4.46618 2.86982 3.16452 3.30019 6.11446 4.99082 34 c - - - 2.68618 4.42225 2.77519 2.73123 3.46354 2.40513 3.72494 3.29354 2.67741 2.69355 4.24690 2.90347 2.73739 3.18146 2.89801 2.37887 2.77519 2.98518 4.58477 3.61503 0.01490 4.60991 5.33226 0.61958 0.77255 0.48576 0.95510 35 1.57064 4.56331 4.08992 3.97275 5.06626 0.70533 5.00881 4.49954 4.13556 4.21770 5.03115 3.91925 4.14760 4.34480 4.35117 2.83639 3.19077 3.78941 6.37913 5.26062 35 g - - - 2.68618 4.42225 2.77519 2.73123 3.46354 2.40513 3.72494 3.29354 2.67741 2.69355 4.24690 2.90347 2.73739 3.18146 2.89801 2.37887 2.77519 2.98518 4.58477 3.61503 0.01490 4.60991 5.33226 0.61958 0.77255 0.48576 0.95510 36 3.54970 4.76667 5.56976 5.11867 4.05357 5.10508 5.86896 0.69955 5.04243 2.46062 3.79583 5.28214 5.35270 5.29513 5.20862 4.54982 3.81805 1.37129 6.18564 4.97377 36 i - - - 2.68618 4.42225 2.77519 2.73123 3.46354 2.40513 3.72494 3.29354 2.67741 2.69355 4.24690 2.90347 2.73739 3.18146 2.89801 2.37887 2.77519 2.98518 4.58477 3.61503 0.01490 4.60991 5.33226 0.61958 0.77255 0.48576 0.95510 37 0.33153 4.75021 4.40686 4.30261 4.98231 3.54503 5.20354 4.24369 4.34423 4.07997 5.06373 4.20507 4.35385 4.61429 4.49324 3.11385 3.44352 3.74593 6.31351 5.23450 37 A - - - 2.68618 4.42225 2.77519 2.73123 3.46354 2.40513 3.72494 3.29354 2.67741 2.69355 4.24690 2.90347 2.73739 3.18146 2.89801 2.37887 2.77519 2.98518 4.58477 3.61503 0.01490 4.60991 5.33226 0.61958 0.77255 0.48576 0.95510 38 2.57216 1.10600 4.57787 4.33033 4.68803 3.31133 5.01577 4.07698 4.20861 3.85895 4.71748 4.03315 4.12886 4.43868 4.33993 1.16175 3.11457 3.51325 6.10347 4.94639 38 c - - - 2.68618 4.42225 2.77519 2.73123 3.46354 2.40513 3.72494 3.29354 2.67741 2.69355 4.24690 2.90347 2.73739 3.18146 2.89801 2.37887 2.77519 2.98518 4.58477 3.61503 0.01490 4.60991 5.33226 0.61958 0.77255 0.48576 0.95510 39 1.13529 4.49155 4.13466 3.89596 4.90876 3.27530 4.87158 4.33040 3.93767 4.04272 4.84767 3.84731 4.08319 4.18051 4.18377 0.98778 3.09403 3.66106 6.25341 5.08965 39 s - - - 2.68618 4.42225 2.77519 2.73123 3.46354 2.40513 3.72494 3.29354 2.67741 2.69355 4.24690 2.90347 2.73739 3.18146 2.89801 2.37887 2.77519 2.98518 4.58477 3.61503 0.01490 4.60991 5.33226 0.61958 0.77255 0.48576 0.95510 40 1.35958 4.43653 4.40471 3.86808 3.62801 3.96603 4.51964 2.49530 3.75624 2.47164 2.19214 4.06496 4.41884 4.00472 3.96781 3.30869 3.20019 1.74965 5.25305 4.06522 40 a - - - 2.68618 4.42225 2.77519 2.73123 3.46354 2.40513 3.72494 3.29354 2.67741 2.69355 4.24690 2.90347 2.73739 3.18146 2.89801 2.37887 2.77519 2.98518 4.58477 3.61503 0.01490 4.60991 5.33226 0.61958 0.77255 0.48576 0.95510 41 2.87019 5.08680 3.14017 1.45337 2.57281 3.63263 3.82268 3.75587 2.55518 3.32169 4.16068 3.15759 4.04164 2.98311 2.13275 2.88780 3.10016 3.43427 5.48640 4.11555 41 e - - - 2.68618 4.42225 2.77519 2.73123 3.46354 2.40513 3.72494 3.29354 2.67741 2.69355 4.24690 2.90347 2.73739 3.18146 2.89801 2.37887 2.77519 2.98518 4.58477 3.61503 0.01005 4.60506 * 0.61958 0.77255 0.00000 * //
What's important to note is that while the model is built by using information from the original sequences, the original sequences themselves do not appear anywhere in the model. While the models that are used to generate AI art are far more complicated than this simple example, that remains true. No copies of any artwork are stored in the model.
But, if the AI models do not contain copies of the original source materials, then how come AI sometimes replicates existing artwork? Before we explain that, it's worth remembering that extremely similar images are not exclusive to AI. Sometimes images might look the same because one is a parody of another or because of a set style, such as a still life of a bowl of fruit. Images may also look the same when they both contain the same, particularly dramatic element or because there are only a limited number of ways to structure a composition. For example, if one has two opposing groups and wishes to capture all the members in one image, it's not unlikely to come up with the same composition as is used in both the Cats & Dogs and Captain America: Civil War posters. It is just one of several extremely-similar promotional images.
One of the big differences between human artists and AI (as well as one of the limitations), is that AI does not have any conceptual understanding of what it is drawing. If a human artist were to draw a fox, they know that a fox is an animal, that there are different species of fox, the basic body shape of a fox, how the limbs move, how the body changes during growth and so on. Such a conceptual understanding will allow the artist to imagine how a fox might look in different situations. AI can not do that.
In general, if AI art is copying pieces of the training data -- not general concepts and styles but individual works of art -- then it's a bad model and should be fixed! The solution is typically to train on more data, so the model captures less of each individual image.
— Daniel Lowd (@dlowd) January 4, 2023
Lacking conceptual knowledge or any sort of background knowledge which humans enjoy, AI models are limited to what they are given in their training data. They have to pull out the pattern which repeats in all of the training images, the same way that our HMM above knew that the multiple protein sequences were of the same protein and had to describe what they had in common. The fewer training images that are used, the greater the influence of any one particular image in the final model.
That applies both to the training set as a whole and to any particular label. If there is only one image of a horse in the training data, then any horse images produced by that model will be very similar, albeit not identical, to the training image. Ironically, demands to exclude artworks from training data sets due to fears of unauthorised copies stemming from image duplication actually make it more likely for duplications to occur.
Additionally, AI art is generated in response to text prompts. Given a small training set, it's possible that a prompt will be similar enough to the description of a specific training image that the majority of AI-generated images will use the part of the model that was trained on that one picture. Similarity due to text prompts should not be surprising, as this is how commissioners and artists communicate too. The more specific the prompt, the more similar artworks will be, even from artists working independently. If I ask 100 artists to draw "a fox," the images will probably include all sorts of species, poses and settings. If I ask 100 artists to draw "a fennec fox sitting on a rock with a sunset in the background," I would not be surprised to get back several pictures which look extremely similar.
Finally, one may have seen the following set of comparisons being shared as evidence that even AIs like Stable Diffusion, which has been trained on large datasets, save and reproduce exact copies of images.
The picture is taken from a pre-print that was trying to understand when and why AI produces copies of the training data. In addition to the already described problem of text prompt similarity, it has identified issues with the training data set, including overfitting as likely causes. Importantly, these are technical problems which can easily be addressed. Conversely the similarities in pictures does not suggest that the model is storing copies of specific artworks:
We speculate that replication behavior in Stable Diffusion arises from a complex interaction of factors, which include that it is text (rather than class) conditioned, it has a highly skewed distribution of image repetitions in the training set, and the number of gradient updates during training is large enough to overfit on a subset of the data.
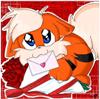
About the author
Rakuen Growlithe — read stories — contact (login required)a scientist and Growlithe from South Africa, interested in science, writing, pokemon and gaming
I'm a South African fur, originally from Cape Town. I'm interested in science, writing, gaming, all sorts of furry stuff, Pokemon and some naughtier things too! I've dabbled in art before but prefer writing. You can find my fiction on SoFurry and non-fiction on Flayrah.
Comments
Always good to know how things function.
The grey area I think here is that, even if the images aren't stored, the data of the image is processed by a program in a way that the original artist may not have intended when they decided to share it with the internet.
Different artists have differing boundaries on how they wish their art to be utilized. And regardless of "how" the program is utilizing their work, whether just referencing rather than copying, the artist is going to feel they should have some say in it regardless.
Going to be interesting if we start seeing an art program show up that can interlace a sort of DRM into the metadata to cause it to be misinterpreted by algorithms scrapping meta data in the future.
I saw it as maybe a door opener for large art software developers to incorporate creator protections into their software. You know, something a small artist may be happy to pay a subscription for. But instead they seem to be moving the opposite way. Clearly there is a vocal demand, it's a waste if no one at least attempts to oblige.
On a technical level that's possible. There was some way to use image noise and changes that were too subtle for humans to see to fool image recognition into confusing pictures of one thing for something else. But, as I will argue, there is wider context for these discussions and I think such moves on restricting art use are not beneficial to society at large or to artists.
"If all mankind minus one, were of one opinion, and only one person were of the contrary opinion, mankind would be no more justified in silencing that one person, than he, if he had the power, would be justified in silencing mankind."
~John Stuart Mill~
By "Future" I mean in a month apparently: https://twitter.com/NymnWales/status/1636428437010419712?s=20
Scientifically, this is article is not entirely correct.
The simplest way to imagine how these NN work is to think about Eigenimages that are linked to the latent vectors. In most cases these Eigenimages will be a statistical average of many examples for a particular keyword / vector in latent space. However if you don't have enough examples, you will end up with basically a copy (just modified/compressed through the decomposition into edges / shapes / etc. that the convolutional layer imposes) due to overtraining of the NN.
Here is a paper from last year that looked into the image replication issue for stable diffusion https://arxiv.org/pdf/2212.03860.pdf
The question then becomes if it is even possible to avoid that kind of image replication issue - and I think it will be very hard to keep the model from choosing certain "eigenimages" for the internal representation within the weights.
There are just too high correlations for certain keywords (like scream and the Munch painting) to prevent that sensibly. In other cases it is probably impossible to increase coverage for certain unique keywords, which might only be connected to a single image.
In addition the computational (and cost) requirements of a "fresh" training are so high, that many techniques to prevent overfitting are not easily applicable.
That's why I doubt the "technical problems which can easily be addressed" is a correct statement.
"What's important to note is that while the model is built by using information from the original sequences, the original sequences themselves do not appear anywhere in the model."
The same is true for JPEG compression - when comparing them to some bit pattern from eg. a PNG file. Both JPEG compression and the convolutional layers of a NN translate images into some "transform domain" (in a lossy way) - and the actual data is stored in that format. For NN it's "stored" in the weights in a statistical way (with some more losses) but as the paper shows, it's still possible to reproduce statistically significant images afterwards in an recognizable way.
Let me ask this then. What's the difference between what's being done with AI image generators (I won't call what they make art), vs a human artist using an existing image as a reference? The latter never ask either. If it's ok for the latter to do it with a few images, then the overwhelming issue seems to be the scale of the number of images used to train the generators. I don't think that's enough to make one ok and the other not ok.
Post new comment